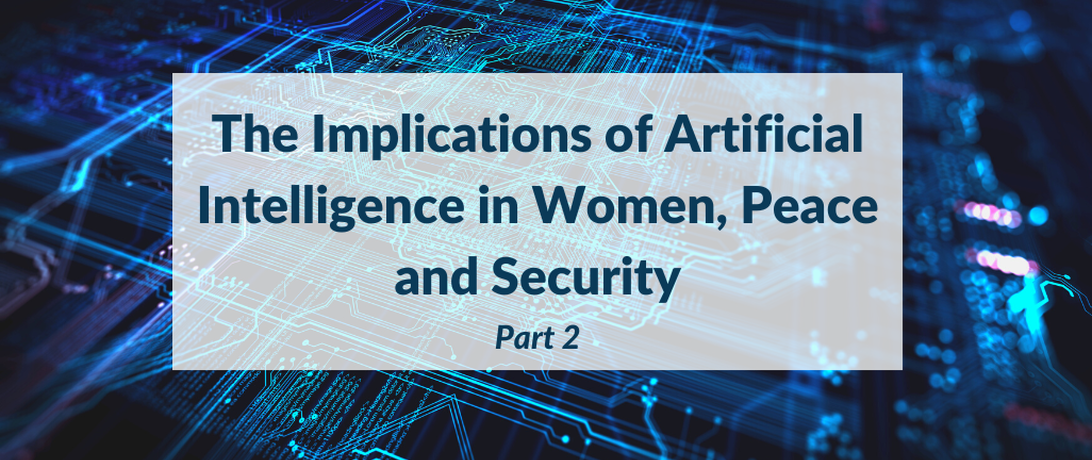
A review of the United Nations Institute for Disarmament Research recent report, "Does Military AI Have Gender?: Understanding bias and promoting ethical approaches in military applications of AI."
AI in Practice
Though computer systems are increasingly replacing real people, these systems still require many human actors to build, implement and maintain them. Computer and data science, technology, and cybersecurity fields also reflect systemic inequalities. Women and other minorities have been, and remain, excluded from technology sectors - in the US today, the tech industry is 75% male and 70% white. Global inequalities are mapped onto the digital ecosystem - only 58% of the world has internet access (most of which is in the global north), and, furthermore, the hardware and resources required to build advanced AI systems are incredibly expensive and require a lot of energy. Data collection similarly requires financial and human capital that most countries don’t possess. African and Latin American countries, for example, account for less than 5% of the world’s data collection centers, which means populations from these areas are underrepresented in data and are not in control of how data tied to their population is analyzed and encoded into machine learning systems.
Gender and Military AI
Chandler emphasizes the conflict, peace, and security context, and how AI systems have unintentionally disrupted progress made by the WPS agenda in recent years. Military recruitment algorithms are more likely to pass overqualified women candidates - especially given historically low levels of female participation. A voice control system might not recognize the voice of a female pilot. A 2016 study showed voice recognition tools are 70% more likely to accurately recognize a man’s voice over a woman's. Automated emergency relief systems may overlook the specific needs of women and girls.
Gender bias is prevalent in the context of Autonomous Weapons Systems (AWS). These systems (and their human operators) search for, and engage, targets based on programmed descriptions - more or less predicated on gendered notions of who a ‘threat.’ As traditional gender norms assume men to be the ‘violent,’ warriors, AWS systems frequently mis-categorize civilian males as combatants - leading to more civilian casualties. In Afghanistan, for example, where drone warfare was heavily scrutinized for its disproportionate impact on civilians, ‘military targets’ were identified as any military-aged male. Furthermore, data sets from prior conflicts are recalled and used in new contexts - overlooking context-specific gender, age, uniform (dress), and weapons, reflecting AI’s output are limited by ‘recognizable’ patterns. This illuminates how traditional gender norms harm men as well as women.
Despite the 2019 Conventional Weapons Conventions (CCW) framework that asserts AWS must fit within a responsible chain of human command and control, it often does not matter if the user chooses to protect civilians if they are not adequately represented.
The ongoing conflict in Libya, as Chandler discusses, revealed a new type of “kamikaze” AWS that “hunted down and remotely engaged,” retreating opposition forces. The machine reportedly was capable of “selecting and engaging human targets based on machine-learning object classification”- essentially denoting based on proximity to an object person. It was unclear whether there was a remote operator. Though there is little information on these drones (used to deflect criticism), Chandler connects the rise of drone warfare and “battlefield experiments” to Libya’s humanitarian crisis, war crimes, and rising civilian casualties.
These “Kamikaze” style drones are reportedly being used by Russia on civilian targets in Ukraine, revealing how this technology will become more and more prevalent in the future of conflict and warfare and how it can have devastating implications for civilian populations - exacerbating insecurity and humanitarian crisis.
Towards an ‘Ethical AI:’ AI and the Women, Peace, and Security Agenda
As military AI increasingly becomes the norm in national security strategy, policy, and practice, the WPS agenda and gender mainstreaming should be incorporated into all stages of development and application. A gender-sensitive approach would consider: “How are women’s participation and representation accounted for by military AI? Has conflict prevention been considered in the system design? Does the model provide for the protection of human rights, and do these protections apply equally to women, men, girls, and boys? How would post-conflict recovery missions be addressed by military AI?”
Chandler asserts that, as militaries around the world integrate machine learning processes into the armed forces, they need to make commitments to ‘ethical AI’ and address bias against gender and its intersection with race, ethnicity, age, and ability. Independent gender-based audits of data sets and algorithms could reveal biases within algorithms and machine learning models. Information about the motivations, processes and intended uses of data sets needs to be analyzed and made available, and systems should be evaluated using testing scenarios distinct from data sets used to train the model. Members of the evaluation team should be diverse and interdisciplinary - and should at a minimum include a general expert. Users, too, need to be trained to recognize biases and understand how it is factored into every stage of AI development. AI does have the potential to be a system of accountability for war and humanitarian crimes and a positive tool for gender mainstreaming in conflict, interventions, and relief and recovery. However, we need to ensure protections and accountability measures apply equally - and are attuned to the specific needs of - men, women, boys, and girls.
Check out Part 1 of the Implications of Artificial Intelligence in Women, Peace and Security.
Read more about Our Secure’s Future research on the digital ecosystem at our Project Delphi page.
Article Details
Published
Written by
Topic
Program
Content Type
Opinion & Insights